Reports of Death of Moore’s Law Are Greatly Exaggerated as AI Expands
By John P. Desmond, AI Trends Editor Moore’s Law is far from dead and in fact, we are entering a new era of innovation, thanks to a combination of newly-developed specialized chips combined with the march of AI and machine learning. “These unprecedented and massive improvements in processing power combined with data and artificial intelligence […]
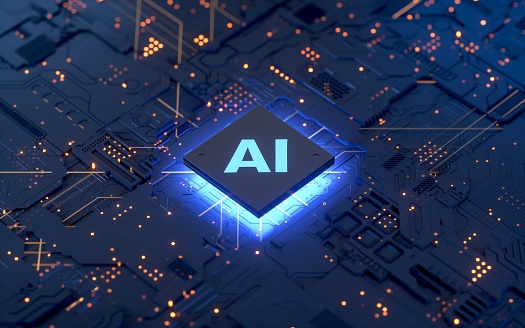
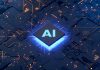
By John P. Desmond, AI Trends Editor
Moore’s Law is far from dead and in fact, we are entering a new era of innovation, thanks to a combination of newly-developed specialized chips combined with the march of AI and machine learning.
“These unprecedented and massive improvements in processing power combined with data and artificial intelligence will completely change the way we think about designing hardware, writing software and applying technology to businesses,” suggests a recent account from siliconAngle written by Dave Vellante and David Floyer.
Vellante is the co-CEO of SiliconAngle Media and a long-time tech industry analyst. David Floyer worked more than 20 years at IBM and later at IDC, where he worked on IT strategy.
Moore’s Law, a prediction made by American engineer Gordon Moore in 1965, called for a 40% performance improvement in central processing year to year, based on the number of transistors per silicon chip doubling every year.
However, the explosion of alternative processing power in the form of new systems on a chip (SoC) is increasing dramatically faster, at the rate of 100% per year, the authors suggest. Using as an example Apple’s SoC developments from the A9 to the A14 five-nanometer Bionic system on a chip, the authors say improvements since 2015 have been on a pace higher than 118% annually.
This has translated to powerful new AI on iPhones that include facial recognition, speech recognition, language processing, rendering videos, and augmented reality.
With processing power accelerating and the cost of chips decreasing, the bottlenecks emerging are in storage and networks as processing—the authors suggest 99%—is being pushed to the edge, where most data is originated.
“Storage and networking will become increasingly distributed and decentralized,” the authors stated, adding, “With custom silicon and processing power placed throughout the system with AI embedded to optimize workloads for latency, performance, bandwidth, security, and other dimensions of value.”
These massive increases in processing power and cheaper chips will power the next wave of AI, machine intelligence, machine learning, and deep learning. And while much of AI today is focused on building and training models, mostly happening in the cloud, “We think AI inference will bring the most exciting innovations in the coming years.”
To perform inferencing, the AI uses a trained machine learning algorithm to make predictions, and with local processing its training is applied to make micro-adjustments in real time. “The opportunities for AI inference at the edge and in the “internet of things” are enormous,” the authors stated.
The use of AI inferencing will be on the rise as it is incorporated into autonomous cars, which learn as they drive, smart factories, automated retail, intelligent robots, and content production. Meanwhile, AI applications based on modeling, such as fraud detection and recommendation engines, will remain important but not see the same increasing rates of use.
“If you’re an enterprise, you should not stress about inventing AI,” the authors suggest. “Rather, your focus should be on understanding what data gives you competitive advantage and how to apply machine intelligence and AI to win.”
AI Hardware Innovations
The trend in more powerful AI processors is good for the semiconductor and electronics industry. Five innovations in AI hardware point to the trend, according to a recent account in eletimes.
AI in Quantum Hardware. IBM has the Q quantum computer designed and built for commercial use, Google has pursued quantum chips with the Foxtail, Bristlecone, and Sycamore projects.
Application Specific Integrated Circuits (ASICs) are designed for a particular use, such as run voice analysis or bitcoin mining.
Programmable Gate Arrays are integrated circuits for design configuration and customer needs in the manufacturing process. It works as a field-oriented mechanism and compares to semiconductor devices based around a configurable matrix.
Neuromorphic Chips are designed with artificial neurons and synapses that mimic the activity of the human brain, and aim to identify the shortest route to solving problems.
AI in Edge Computing Chips are capable of conducting an analysis with no latency, a favored choice for applications where data bandwidth is paramount, such as CT scan diagnostics.
AI Software Company Determined AI Aims to Unlock Value
Startup companies are embedding AI into their software in order to help customers unlock the value of AI for their own organizations.
One example is Determined AI, founded in 2017 to offer a deep learning training platform to help data scientists train better models.
Before founding Determined AI, CEO and cofounder Evan Sparks was a researcher at the AmpLab at UC Berkeley, where he focused on distributed systems for large-scale machine learning, according to a recent account in ZDNet. He worked at Berkeley with David Patterson, a computer scientist who was arguing that custom silicon was the only hope for continued computer processing growth needed to keep pace with Moore’s Law.
Determined AI has developed a software layer, called ONNX (Open Neural Network Exchange), that sits underneath an AI development tool such as TensorFlow or PyTorch, and above a range of AI chips that it supports. ONNX originated within Facebook, where developers sought to have AI developers do research in whatever language they chose, but to always deploy in a consistent framework.
“Many systems are out there for preparing your data for training, making it high performance and compact data structures and so on,” Sparks stated. “That is a different stage in the process, different workflow than the experimentation that goes into model training and model development.”
“As long as you get your data in the right format while you’re in model development, it should not matter what upstream data system you’re doing,” he suggested. “Similarly, as long as you develop in these high-level languages, what training hardware you’re running on, whether it’s GPUs or CPUs or exotic accelerators should not matter.”
This might also provide a path toward controlling the cost of AI development. “You let the big guys, the Facebooks and the Googles of the world do the big training on huge quantities of data with billions of parameters, spending hundreds of GPU years on a problem,” Sparks stated. “Then instead of starting from scratch, you take those models and maybe use them to form embeddings that you’re going to use for downstream tasks.”
This could streamline some natural language processing and image recognition applications, for example.
Read the source articles in siliconAngle, in eletimes and in ZDNet.