What is vector search? Better search through AI
Suppose you wanted to implement a music service that would behave like Spotify, finding you songs that were similar to ones you like. How would you go about it? One way would be to classify each song by a number of characteristics, save those “vectors” in an indexed database, and search the database to find the song-description vectors “near” your favorites. In other words, you could perform a vector similarity search. [ Also on InfoWorld: How to choose a cloud machine learning platform ] What is vector similarity search? There are typically four components to vector similarity search: vector embeddings that capture the key characteristics of the original object, such as a song, an image, or some text; distance metrics that represent “nearness” between vectors; search algorithms; and a database that holds the vectors and supports the vector search with indexes.To read this article in full, please click here
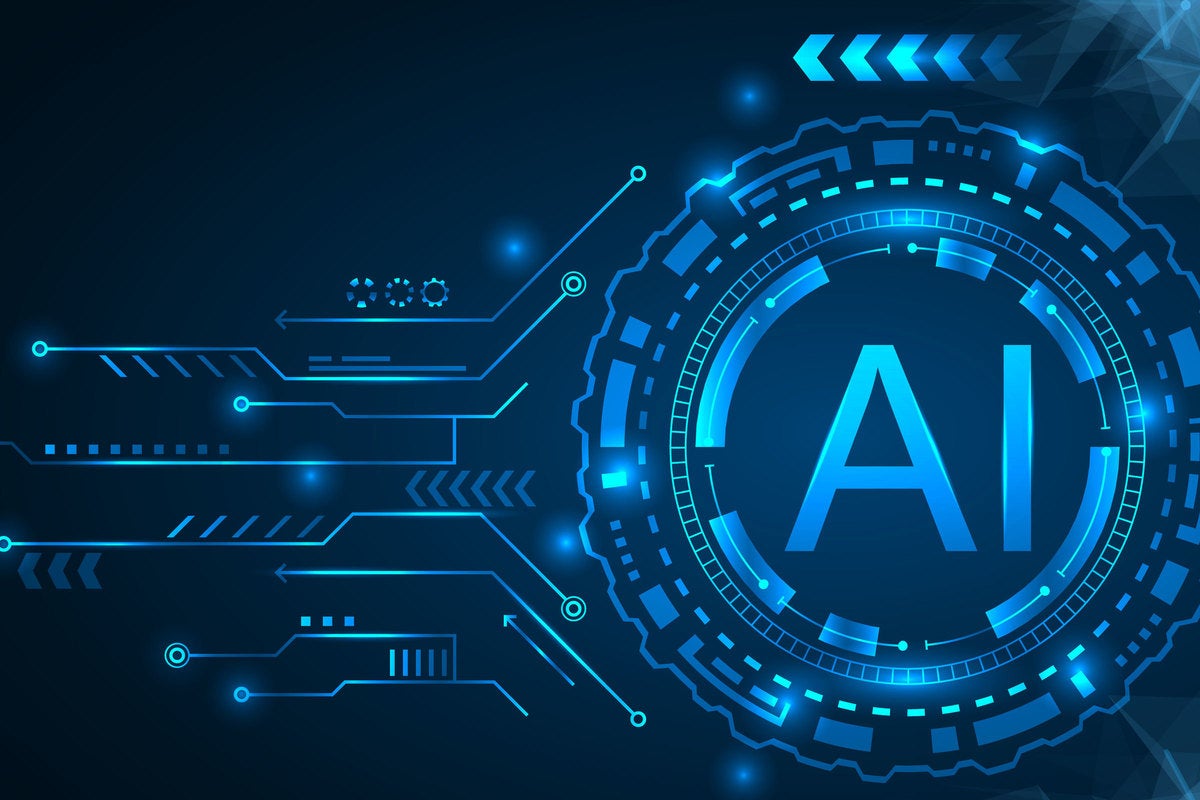
Suppose you wanted to implement a music service that would behave like Spotify, finding you songs that were similar to ones you like. How would you go about it? One way would be to classify each song by a number of characteristics, save those “vectors” in an indexed database, and search the database to find the song-description vectors “near” your favorites. In other words, you could perform a vector similarity search.
What is vector similarity search?
There are typically four components to vector similarity search: vector embeddings that capture the key characteristics of the original object, such as a song, an image, or some text; distance metrics that represent “nearness” between vectors; search algorithms; and a database that holds the vectors and supports the vector search with indexes.