What is neural architecture search? AutoML for deep learning
Neural architecture search is the task of automatically finding one or more architectures for a neural network that will yield models with good results (low losses), relatively quickly, for a given dataset. Neural architecture search is currently an emergent area. There is a lot of research going on, there are many different approaches to the task, and there isn’t a single best method generally — or even a single best method for a specialized kind of problem such as object identification in images.Neural architecture search is an aspect of AutoML, along with feature engineering, transfer learning, and hyperparameter optimization. It’s probably the hardest machine learning problem currently under active research; even the evaluation of neural architecture search methods is hard. Neural architecture search research can also be expensive and time-consuming. The metric for the search and training time is often given in GPU-days, sometimes thousands of GPU-days.To read this article in full, please click here
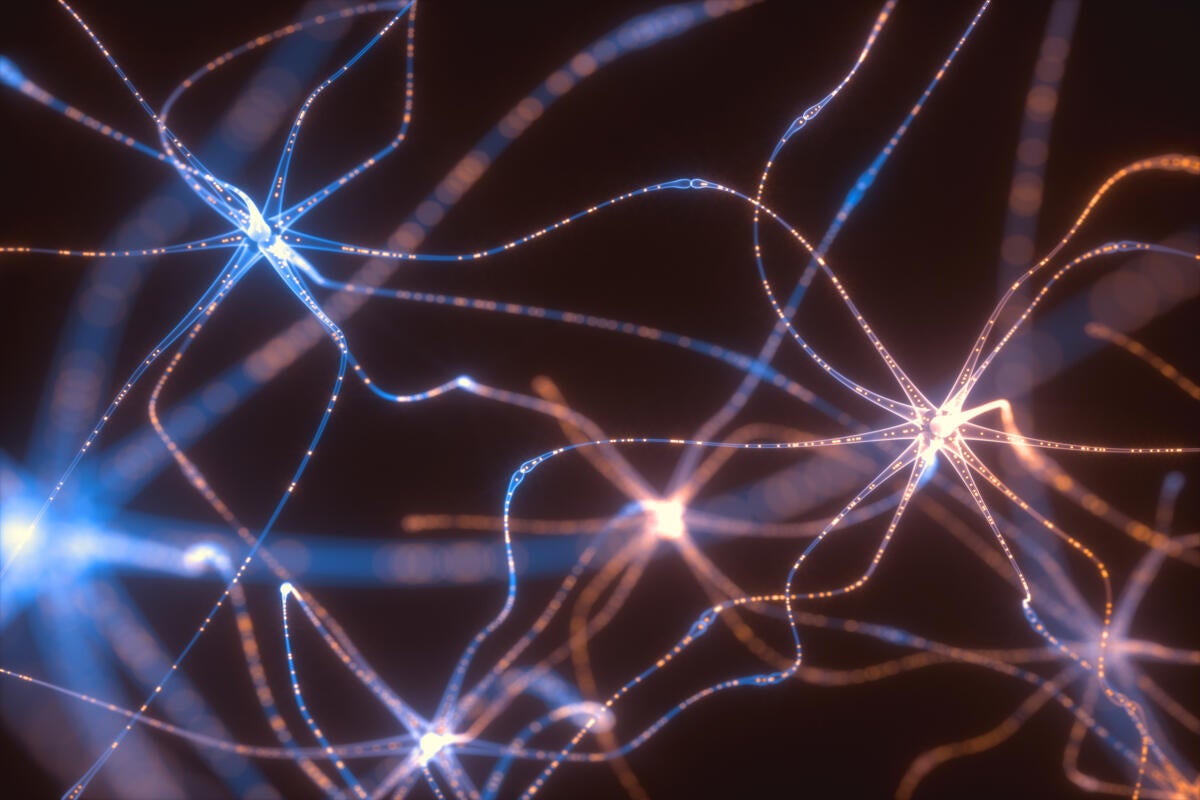
Neural architecture search is the task of automatically finding one or more architectures for a neural network that will yield models with good results (low losses), relatively quickly, for a given dataset. Neural architecture search is currently an emergent area. There is a lot of research going on, there are many different approaches to the task, and there isn’t a single best method generally — or even a single best method for a specialized kind of problem such as object identification in images.
Neural architecture search is an aspect of AutoML, along with feature engineering, transfer learning, and hyperparameter optimization. It’s probably the hardest machine learning problem currently under active research; even the evaluation of neural architecture search methods is hard. Neural architecture search research can also be expensive and time-consuming. The metric for the search and training time is often given in GPU-days, sometimes thousands of GPU-days.