The Age of Artificial Intelligence: AI Chips to 2034
Artificial Intelligence is transforming the world as we know it; from the success of DeepMind over Go world champion Lee Sedol in 2016 to the robust predictive abilities of OpenAI’s ChatGPT, the complexity of AI training algorithms is growing at a startlingly fast pace, where the amount of compute necessary to run newly-developed training algorithms […] The post The Age of Artificial Intelligence: AI Chips to 2034 appeared first on Edge AI and Vision Alliance.
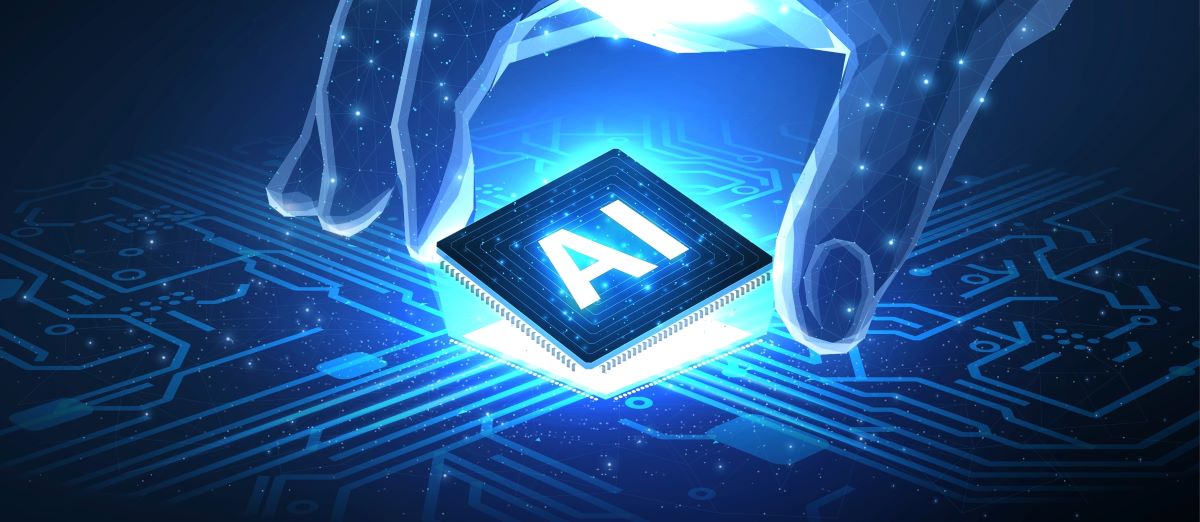
Artificial Intelligence is transforming the world as we know it; from the success of DeepMind over Go world champion Lee Sedol in 2016 to the robust predictive abilities of OpenAI’s ChatGPT, the complexity of AI training algorithms is growing at a startlingly fast pace, where the amount of compute necessary to run newly-developed training algorithms appears to be doubling roughly every four months. In order to keep pace with this growth, hardware for AI applications is needed that is not just scalable – allowing for longevity as new algorithms are introduced while keeping operational overheads low – but is also able to handle increasingly complex models at a point close to the end-user.
Drawing from the “AI Chips: 2023-2033” and “AI Chips for Edge Applications 2024-2034: Artificial Intelligence at the Edge” reports, IDTechEx predicts that the growth of AI, both for training and inference within the cloud and inference at the edge, is due to continue unabated over the next ten years, as our world and the devices that inhabit them become increasingly automated and interconnected.
The why and what of AI chips
The notion of designing hardware to fulfill a certain function, particularly if that function is to accelerate certain types of computations by taking control of them away from the main (host) processor, is not a new one; the early days of computing saw CPUs (Central Processing Units) paired with mathematical coprocessors, known as Floating-Point Units (FPUs). The purpose was to offload complex floating point mathematical operations from the CPU to this special-purpose chip, as the latter could handle computations more efficiently, thereby freeing the CPU up to focus on other things.
As markets and technology developed, so too did workloads, and so new pieces of hardware were needed to handle these workloads. A particularly noteworthy example of one of these specialized workloads is the production of computer graphics, where the accelerator in question has become something of a household name: the Graphics Processing Unit (GPU).
Just as computer graphics required the need for a different type of chip architecture, the emergence of machine learning has brought about a demand for another type of accelerator, one that is capable of efficiently handling machine learning workloads. Machine learning is the process by which computer programs utilize data to make predictions based on a model and then optimize the model to better fit with the data provided, by adjusting the weightings used. Computation, therefore, involves two steps: Training and Inference.
The first stage of implementing an AI algorithm is the training stage, where data is fed into the model, and the model adjusts its weights until it fits appropriately with the provided data. The second stage is the inference stage, where the trained AI algorithm is executed, and new data (not provided in the training stage) is classified in a manner consistent with the acquired data.
Of the two stages, the training stage is more computationally intense, given that this stage involves performing the same computation millions of times (the training for some leading AI algorithms can take days to complete). As such, training takes place within cloud computing environments (i.e. data centers), where a large number of chips are used that can perform the type of parallel processing required for efficient algorithm training (CPUs process tasks in a serialized manner, where one execution thread starts once the previous execution thread has finished. In order to minimize latency, large and numerous memory caches are utilized so that most of the execution thread’s running time is dedicated to processing. By comparison, parallel processing involves multiple calculations occurring simultaneously, where lightweight execution threads are overlapped such that latency is effectively masked. Being able to compartmentalize and carry out multiple calculations simultaneously is a major benefit for training AI algorithms, as well as in many instances of inference). By contrast, the inference stage can take place within both cloud and edge computing environments. The aforementioned reports detail the differences between CPU, GPU, Field Programmable Gate Array (FPGA) and Application-Specific Integrated Circuit (ASIC) architectures, and their relative effectiveness in handling machine learning workloads.
Within the cloud computing environment, GPUs currently dominate and are predicted to continue to do so over the next ten-year period, given Nvidia’s dominance in the AI training space. For AI at the edge, ASICs are preferred, given that chips are more commonly designed with specific problems in mind (such as for object detection within security camera systems, for example). As the below graph shows, Digital Signal Processors (DSPs) also account for a significant share of AI coprocessing at the edge, though it should be noted that this large figure is primarily due to Qualcomm‘s Hexagon Tensor Processor (which is found in their modern Snapdragon products) being a DSP. Should Qualcomm redesign the HTP such that it strays from being a DSP, then the forecast would heavily skew in favour of ASICs.
Revenue generated by different chip architectures for edge devices, 2024 to 2034.
AI as a driver for semiconductor manufacture
Chips for AI training are typically manufactured at the most leading-edge nodes (where nodes refer to the transistor technology used in semiconductor chip manufacture), given how computationally intensive the training stage of implementing an AI algorithm is. Intel, Samsung, and TSMC are the only companies that can produce 5 nm node chips. Out of these, TSMC is the furthest along with securing orders for 3 nm chips. TSMC has a global market share for semiconductor production that is currently hovering at around 60%. For the more advanced nodes, this is closer to 90%. Of TSMC’s six 12-inch fabs and six 8-inch fabs, only two are in China, and one is in the USA. The rest are in Taiwan. The semiconductor manufacture part of the global supply chain is therefore heavily concentrated in the APAC region, principally Taiwan.
Such a concentration comes with a great deal of risk should this part of the supply chain be threatened in some way. This is precisely what occurred in 2020 when a number of complementing factors (discussed further in the “AI Chips: 2023 – 2033” report) led to a global chip shortage. Since then, the largest stakeholders (excluding Taiwan) in the semiconductor value chain (the US, the EU, South Korea, Japan, and China) have sought to reduce their exposure to a manufacturing deficit, should another set of circumstances arise that results in an even more exacerbated chip shortage. This is shown by the government funding announced by these major stakeholders in the wake of the global chip shortage, represented below.
Funding as related to government initiatives announced since 2021.
These government initiatives aim to spur additional private investment through the lure of tax breaks and part-funding in the way of grants and loans. While many of the private investments displayed pictorially below were made prior to the announcement of such government initiatives, other additional and/or new private investments have been announced in the wake of them, spurred on as they are by the incentives offered through these initiatives.
Shown here are the proposed and confirmed investments into semiconductor facilities by manufacturers since 2021. Where currencies have been listed in anything but US$, these have been converted to US$ as of May 2023.
A major reason for these government initiatives and additional private spending is the potential of realizing advanced technology, of which AI can be considered. The manufacture of advanced semiconductor chips fuels national/regional AI capabilities, where the possibility for autonomous detection and analysis of objects, images, and speech are so significant to the efficacy of certain products (such as autonomous vehicles and industrial robots) and to models of national governance and security, that the development of AI hardware and software has now become a primary concern for government bodies that wish to be at the forefront of technological innovation and deployment.
Growth of AI chips over the next decade
Revenue generated from the sale of AI chips (including the sale of physical chips and the rental of chips via cloud services) is expected to rise to just shy of USD$300 billion by 2034, at a compound annual growth rate of 22% from 2024 to 2034. This revenue figure incorporates the use of chips for the acceleration of machine learning workloads at the edge of the network, for telecom edge, and within data centers in the cloud. As of 2024, chips for inference purposes (both at the edge and within the cloud) comprise 63% of revenue generated, with this share growing to more than two-thirds of the total revenue by 2034.
This is in large part due to significant growth at the edge and telecom edge, as AI capabilities are harnessed closer to the end-user. In terms of industry vertical, IT & Telecoms is expected to lead the way for AI chip usage over the next decade, with Banking, Financial Services & Insurance (BFSI) close behind, and Consumer Electronics behind that. Of these, the Consumer Electronics industry vertical is to generate the most revenue at the edge, given the further rollout of AI into consumer products for the home. More information regarding industry vertical breakout can be found in the relevant AI reports.
AI chips revenue segmented by purpose.
Revenue generated by AI chips is set to grow at a CAGR of 22% over the next ten years, up to 2034. Of the revenue generated at this time, AI chips for inference purposes will dominate over those used for AI training, as AI migrates more thoroughly to deployment at the edge of the network.
For more information regarding key trends and market segmentations with regards AI chips over the next ten years, please refer to the two reports: “AI Chips: 2023-2033” and “AI Chips for Edge Applications 2024-2034: Artificial Intelligence at the Edge“.
The “AI Chips: 2023-2033” report covers the global AI Chips market across eight industry verticals, with 10-year granular forecasts in seven different categories (such as by geography, by chip architecture, and by application). In addition to the revenue forecasts for AI chips, costs at each stage of the supply chain (design, manufacture, assembly, test & packaging, and operation) are quantified for a leading-edge AI chip. Rigorous calculations are provided, along with a customizable template for customer use, and analyses of comparative costs between leading and trailing edge node chips.
The “AI Chips for Edge Applications 2024-2034: Artificial Intelligence at the Edge” report gives analysis pertaining to the key drivers for revenue growth in edge AI chips over the forecast period, with deployment within the key industry verticals – consumer electronics, industrial automation, and automotive – reviewed. More generally, the report covers the global edge AI Chips market across six industry verticals, with 10-year granular forecasts in six different categories (such as by geography, by chip architecture, and by application).
IDTechEx also offers expert-led data and analysis on these and other related topics through a market intelligence subscription – find out more at www.IDTechEx.com/Subscriptions.
This article is from “Technology Innovations Outlook 2024-2034”, a complimentary magazine of analyst-written articles by IDTechEx providing insights into a number of areas of technology innovation, assessing the landscape now and giving you the outlook for the next decade. You can read the magazine in full at www.IDTechEx.com/Magazine.
IDTechEx guides your strategic business decisions through its Research, Subscription and Consultancy products, helping you profit from emerging technologies. For more information, contact research@IDTechEx.com or visit www.IDTechEx.com.
The post The Age of Artificial Intelligence: AI Chips to 2034 appeared first on Edge AI and Vision Alliance.