Sustainable Strides: How AI and Accelerated Computing Are Driving Energy Efficiency
AI and accelerated computing — twin engines NVIDIA continuously improves — are delivering energy efficiency for many industries. It’s progress the wider community is starting to acknowledge.
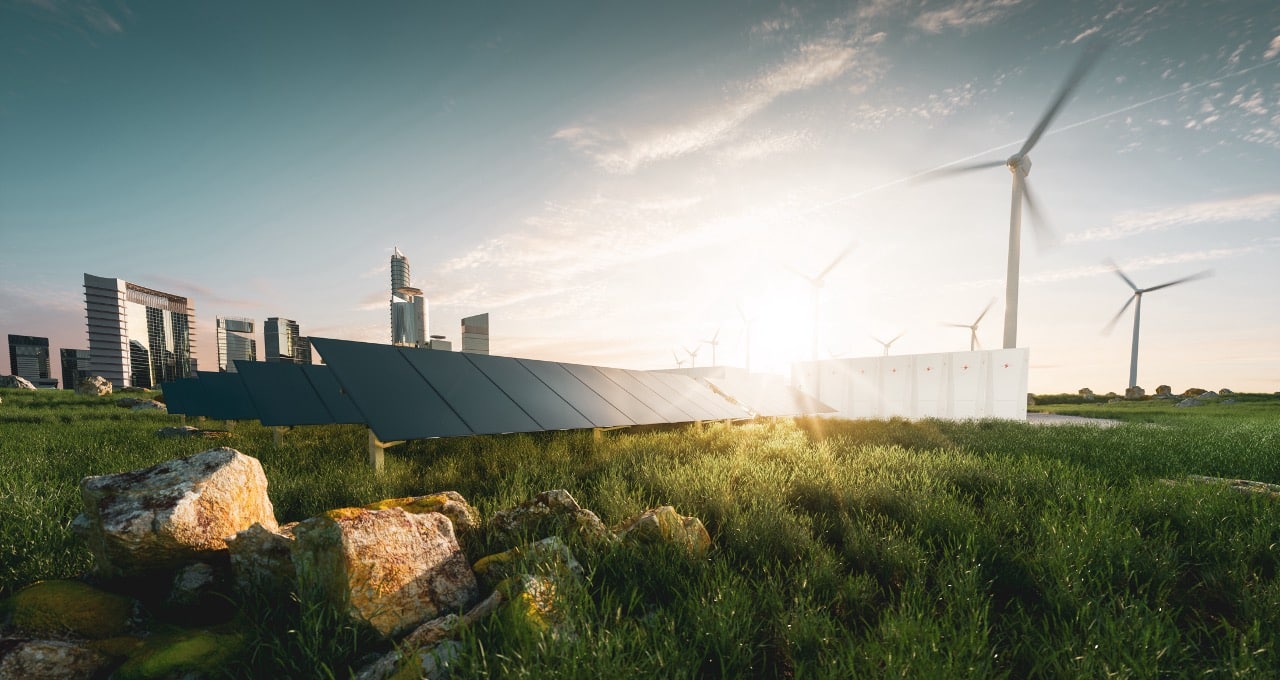
AI and accelerated computing — twin engines NVIDIA continuously improves — are delivering energy efficiency for many industries.
It’s progress the wider community is starting to acknowledge.
“Even if the predictions that data centers will soon account for 4% of global energy consumption become a reality, AI is having a major impact on reducing the remaining 96% of energy consumption,” said a report from Lisbon Council Research, a nonprofit formed in 2003 that studies economic and social issues.
The article from the Brussels-based research group is among a handful of big-picture AI policy studies starting to emerge. It uses Italy’s Leonardo supercomputer, accelerated with nearly 14,000 NVIDIA GPUs, as an example of a system advancing work in fields from automobile design and drug discovery to weather forecasting.
Why Accelerated Computing Is Sustainable Computing
Accelerated computing uses the parallel processing of NVIDIA GPUs to do more work in less time. As a result, it consumes less energy than general-purpose servers that employ CPUs built to handle one task at a time.
That’s why accelerated computing is sustainable computing.
The gains are even greater when accelerated systems apply AI, an inherently parallel form of computing that’s the most transformative technology of our time.
“When it comes to frontier applications like machine learning or deep learning, the performance of GPUs is an order of magnitude better than that of CPUs,” the report said.
By transitioning from CPU-only operations to GPU-accelerated systems, HPC and AI workloads can save over 40 terawatt-hours of energy annually, equivalent to the electricity needs of nearly 5 million U.S. homes.
User Experiences With Accelerated AI
Users worldwide are documenting energy-efficiency gains with AI and accelerated computing.
In financial services, Murex — a Paris-based company with a trading and risk-management platform used daily by more than 60,000 people — tested the NVIDIA Grace Hopper Superchip. On its workloads, the CPU-GPU combo delivered a 4x reduction in energy consumption and a 7x reduction in time to completion compared with CPU-only systems (see chart below).
“On risk calculations, Grace is not only the fastest processor, but also far more power-efficient, making green IT a reality in the trading world,” said Pierre Spatz, head of quantitative research at Murex.
In manufacturing, Taiwan-based Wistron built a digital copy of a room where NVIDIA DGX systems undergo thermal stress tests to improve operations at the site. It used NVIDIA Omniverse, a platform for industrial digitization, with a surrogate model, a version of AI that emulates simulations.
The digital twin, linked to thousands of networked sensors, enabled Wistron to increase the facility’s overall energy efficiency by up to 10%. That amounts to reducing electricity consumption by 120,000 kWh per year and carbon emissions by a whopping 60,000 kilograms.
Up to 80% Fewer Carbon Emissions
The RAPIDS Accelerator for Apache Spark can reduce the carbon footprint for data analytics, a widely used form of machine learning, by as much as 80% while delivering 5x average speedups and 4x reductions in computing costs, according to a recent benchmark.
Thousands of companies — about 80% of the Fortune 500 — use Apache Spark to analyze their growing mountains of data. Companies using NVIDIA’s Spark accelerator include Adobe, AT&T and the U.S. Internal Revenue Service.
In healthcare, Insilico Medicine discovered and put into phase 2 clinical trials a drug candidate for a relatively rare respiratory disease, thanks to its NVIDIA-powered AI platform.
Using traditional methods, the work would have cost more than $400 million and taken up to six years. But with generative AI, Insilico hit the milestone for one-tenth of the cost in one-third of the time.
“This is a significant milestone not only for us, but for everyone in the field of AI-accelerated drug discovery,” said Alex Zhavoronkov, CEO of Insilico Medicine.
This is just a sampler of results that users of accelerated computing and AI are pursuing at companies such as Amgen, BMW, Foxconn, PayPal and many more.
Speeding Science With Accelerated AI
In basic research, the National Energy Research Scientific Computing Center (NERSC), the U.S. Department of Energy’s lead facility for open science, measured results on a server with four NVIDIA A100 Tensor Core GPUs compared with dual-socket x86 CPU servers across four of its key high-performance computing and AI applications.
Researchers found that the apps, when accelerated with the NVIDIA A100 GPUs, saw energy efficiency rise 5x on average (see below). One application, for weather forecasting, logged gains of nearly 10x.
Scientists and researchers worldwide depend on AI and accelerated computing to achieve high performance and efficiency.
In a recent ranking of the world’s most energy-efficient supercomputers, known as the Green500, NVIDIA-powered systems swept the top six spots, and 40 of the top 50.
Underestimated Energy Savings
The many gains across industries and science are sometimes overlooked in forecasts that extrapolate only the energy consumption of training the largest AI models. That misses the benefits from most of an AI model’s life when it’s consuming relatively little energy, delivering the kinds of efficiencies users described above.
In an analysis citing dozens of sources, a recent study debunked as misleading and inflated projections based on training models.
“Just as the early predictions about the energy footprints of e-commerce and video streaming ultimately proved to be exaggerated, so too will those estimates about AI likely be wrong,” said the report from the Information Technology and Innovation Foundation (ITIF), a Washington-based think tank.
The report notes as much as 90% of the cost — and all the efficiency gains — of running an AI model are in deploying it in applications after it’s trained.
“Given the enormous opportunities to use AI to benefit the economy and society — including transitioning to a low-carbon future — it is imperative that policymakers and the media do a better job of vetting the claims they entertain about AI’s environmental impact,” said the report’s author, who described his findings in a recent podcast.
Others Cite AI’s Energy Benefits
Policy analysts from the R Street Institute, also in Washington, D.C., agreed.
“Rather than a pause, policymakers need to help realize the potential for gains from AI,” the group wrote in a 1,200-word article.
“Accelerated computing and the rise of AI hold great promise for the future, with significant societal benefits in terms of economic growth and social welfare,” it said, citing demonstrated benefits of AI in drug discovery, banking, stock trading and insurance.
AI can make the electric grid, manufacturing and transportation sectors more efficient, it added.
AI Supports Sustainability Efforts
The reports also cited the potential of accelerated AI to fight climate change and promote sustainability.
“AI can enhance the accuracy of weather modeling to improve public safety as well as generate more accurate predictions of crop yields. The power of AI can also contribute to … developing more precise climate models,” R Street said.
The Lisbon report added that AI plays “a crucial role in the innovation needed to address climate change” for work such as discovering more efficient battery materials.
How AI Can Help the Environment
ITIF called on governments to adopt AI as a tool in efforts to decarbonize their operations.
Public and private organizations are already applying NVIDIA AI to protect coral reefs, improve tracking of wildfires and extreme weather, and enhance sustainable agriculture.
For its part, NVIDIA is working with hundreds of startups employing AI to address climate issues. NVIDIA also announced plans for Earth-2, expected to be the world’s most powerful AI supercomputer dedicated to climate science.
Enhancing Energy Efficiency Across the Stack
Since its founding in 1993, NVIDIA has worked on energy efficiency across all its products — GPUs, CPUs, DPUs, networks, systems and software, as well as platforms such as Omniverse.
In AI, the brunt of an AI model’s life is in inference, delivering insights that help users achieve new efficiencies. The NVIDIA GB200 Grace Blackwell Superchip has demonstrated 25x energy efficiency over the prior NVIDIA Hopper GPU generation in AI inference.
Over the last eight years, NVIDIA GPUs have advanced a whopping 45,000x in their energy efficiency running large language models (see chart below).
Recent innovations in software include TensorRT-LLM. It can help GPUs reduce 3x the energy consumption of LLM inference.
Here’s an eye-popping stat: If the efficiency of cars improved as much as NVIDIA has advanced the efficiency of AI on its accelerated computing platform, cars would get 280,000 miles per gallon. That means you could drive to the moon on less than a gallon of gas.
The analysis applies to the fuel efficiency of cars NVIDIA’s whopping 10,000x efficiency gain in AI training and inference from 2016 to 2025 (see chart below).
Driving Data Center Efficiency
NVIDIA delivers many optimizations through system-level innovations. For example, NVIDIA BlueField-3 DPUs can reduce power consumption up to 30% by offloading essential data center networking and infrastructure functions from less efficient CPUs.
Last year, NVIDIA received a $5 million grant from the U.S. Department of Energy — the largest of 15 grants from a pool of more than 100 applications — to design a new liquid-cooling technology for data centers. It will run 20% more efficiently than today’s air-cooled approaches and has a smaller carbon footprint.
These are just some of the ways NVIDIA contributes to the energy efficiency of data centers.
Data centers are among the most efficient users of energy and one of the largest consumers of renewable energy.
The ITIF report notes that between 2010 and 2018, global data centers experienced a 550% increase in compute instances and a 2,400% increase in storage capacity, but only a 6% increase in energy use, thanks to improvements across hardware and software.
NVIDIA continues to drive energy efficiency for accelerated AI, helping users in science, government and industry accelerate their journeys toward sustainable computing.
Try NVIDIA’s energy-efficiency calculator to find ways to improve energy efficiency. And check out NVIDIA’s sustainable computing site and corporate sustainability report for more information.