Solve the Right Problems, and Build ‘Anti-Fragile’ AI Systems
By AI Trends Staff As computers have become more advanced and AI has come along to revolutionize software, the amount of data computers are drowning in has also increased. However, having more data does not change how an organization makes a decision. The best path to improved decision-making with the help of AI is not to […]
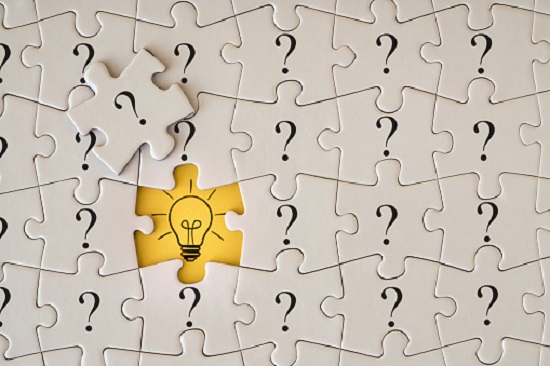
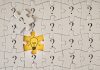
By AI Trends Staff
As computers have become more advanced and AI has come along to revolutionize software, the amount of data computers are drowning in has also increased.
However, having more data does not change how an organization makes a decision. The best path to improved decision-making with the help of AI is not to add more sensors to get more data, it is to improve the communication between stakeholders in the decision, suggests author and data scientist Marianne Bellotti.
“The outcome we’re all hoping for from AI is better decision-making,” stated Bellotti, a data engineer with the US Digital Service, in a recent account in OneZero. She added, “The process of making a decision is less about an objective analysis of data and more about an active negotiation between stakeholders with different tolerances for risk and priorities.”
Lots of effort is expended to clean data for AI. Some estimates are that data scientists spend 80% of their time cleaning data, needed for a centralized AI command center to break down silos to allow interoperable flows for AI models. The Department of Defense spends from $11 billion to $15 billion per year on staff that manages data.
“Do we need to invest billions of dollars cleaning data and sharpening our sensors in order to see benefits from AI?” Bellotti queries.
Discussion of data quality is misleading in that it implies “clean” data is a state where the data is accurate, bias-free, and reusable. However, “Clean is not the same thing as accurate, and accurate is not the same thing as actionable,” Bellotti states.
She adds, “Current AI systems are completely dependent on the quality of their data not because the technology is immature or broken, but because we have designed them to be vulnerable in this fashion. Production AI systems must be designed to be resilient to bad data.”
They can do this by being “anti-fragile,” Bellotti suggests. “In systems thinking, “anti-fragile” is a design that not only recovers from failure but is actually stronger and more effective when exposed to failure,” she states.
The use of AI in medical science has been advancing the quality of decisions from a recognition that many diagnostic challenges have no single correct answer. The process of diagnosing a patient includes a cycle of defining assumptions, ordering tests and narrowing the set of possible answers further and further until a solution is found.
“When the goal of A.I. is not to best the top experts but instead reinforce and support good decision-making practices, that technology is resilient to bad data and capable of becoming anti-fragile,” states Bellotti, who is also author of the book, Kill It with Fire (No Starch Press, 2021) about challenges around modernizing legacy computer systems.
Algorithms Good at Specific Tasks, Not Generalizing
In a similar vein, while AI continues to grow with algorithms that can perform specific tasks, they are not able to generalize their capabilities beyond their narrow domains, suggests the author of the book, Algorithms are Not Enough. (MIT Press, 2020)
“The intellectual tasks, such as chess playing, chemical structure analysis, and calculus are relatively easy to perform with a computer. Much harder are the kinds of activities that even a one-year-old human or a rat could do,” states author Herbert L. Roitblat, principal data scientist at Mimecast, in an account from TechTalks.
Humans play a critical role in deciding what problem to solve with AI and keeping the system tuned once it has been fielded. “Human operators must define a specific problem, curate a training dataset, and label the outcomes before they can create a machine learning model,” Roitblat states. “Only when the problem has been strictly represented in its own way can the model start tuning its parameters.”
Well-defined goals can include anomaly detection in cybersecurity and customer segmentation in marketing.
Current AI systems have shortcomings in that they are confined to problems designers are able to structure and simplify. “Artificial intelligence is a work in progress,” Roitblat stated. “Some tasks have advanced further than others. Some have a way to go. The flaws of artificial intelligence tend to be the flaws of its creator rather than inherent properties of computational decision-making.”
Getting to the Goldilocks Problems
In the real world, for a company starting out with AI, picking the first problem to solve is as important as the solution itself. The risk of picking a problem that is too difficult is that the project will fail, and the risk of picking an unimportant problem is that it will not matter if the AI succeeds or not.
In what they call the “classic Goldilocks situation,” the authors of the book Real World AI (Lioncrest Publishing, 2021), state, “You need a problem that is just right. If you can solve the first problem you attack and prove the impact AI can have, you’ll have a much easier time getting support and resources to tackle the next 10 problems,” state authors Alyssa Simpson Rochwerger, director of product management for Blue Shield of California and Wilson Pang, CTO of Appen, in an account on the blog of Appen.
They offer these four suggestions:
Start Small. Problems that involve classifying something into one or two categories are good candidates, while problems that involve resolving ambiguity may not be good candidates. As an example, the software company Autodesk worked on reducing help desk call resolution time. They decided to focus on a narrow problem of password resets; does the customer want one, yes or no. “That was a perfect Goldilocks problem,” the authors stated.
Go Where the Data Is. A large bank of historical data is a good indicator of a potential Goldilocks problem. The Autodesk password-reset effort had a pool of past instances of password-reset inquiries and the corresponding answers from human agents. “All past cases that have been classified into buckets become training data for your model,” the authors state. If a project has too little data available for training, it may not be a good candidate.
Deliver Quick Wins. If a problem can be solved more quickly by using an off-the-shelf AI model, it could be a good Goldilocks candidate for AI. An off-the-shelf model has already been developed; it comes pre-trained; and that data it’s trained on needs to match the problem your organization needs to solve. An example of a currently available model is one that takes incoming customer requests and quickly recognizes the language of the request. Off-the-shelf training datasets are also available.
Make an Impact. Examples include solutions that increase revenue, decrease costs or free up staff from performing mundane or tedious tasks. Also, “A good rule of thumb is to not only be clear on the business impact but be able to clearly measure and prove it,” the authors state. The Autodesk password reset project fit this goal, because it was able to quantify time saved and customer satisfaction scores. Also, if the project is novel or innovative, more workers in the organization will be making suggestions and offering support to the AI team.
Read the source articles in OneZero, TechTalks and on the blog of Appen.