Research Into Large Language Models Hitting Ethical Bumps
By John P. Desmond, AI Trends Editor A research survey of the machine learning community’s dataset collection shows an over-reliance on poorly curated datasets used to train machine learning models. The study authors recommend a culture that cares for the people represented in datasets and respects their privacy and property rights. However, in today’s machine […]
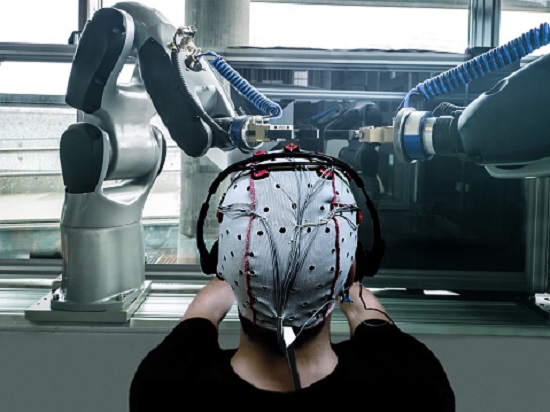
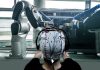
By John P. Desmond, AI Trends Editor
A research survey of the machine learning community’s dataset collection shows an over-reliance on poorly curated datasets used to train machine learning models.
The study authors recommend a culture that cares for the people represented in datasets and respects their privacy and property rights. However, in today’s machine learning environment, “anything goes,” stated the survey authors in an account in VentureBeat.
“Data and its (dis)contents: A survey of dataset development and use in machine learning” was written by University of Washington linguists Amandalynne Paullada and Emily Bender, Mozilla Foundation fellow Inioluwa Deborah Raji, and Google research scientists Emily Denton and Alex Hanna. The paper concluded that large language models contain the capacity to perpetuate prejudice and bias against a range of marginalized communities and that poorly annotated datasets are part of the problem.
Events of the past year have raised the visibility of shortcomings in mainstream datasets that often harm people from marginalized communities. After Timnit Gebru, the AI ethicist, (see coverage in AI Trends) was dismissed from Google in what was reported as “unprecedented research censorship,” the company has started to carry out reviews of research papers on “sensitive topics,” according to an account by Reuters.
The new review procedure asks that researchers consult with legal, policy, and public relations teams before pursuing topics such as face and sentiment analysis and categorizations of race, gender or political affiliation, according to internal web pages explaining the policy.
“Advances in technology and the growing complexity of our external environment are increasingly leading to situations where seemingly inoffensive projects raise ethical, reputational, regulatory, or legal issues,” one of the pages for research staff stated. Reuters could not determine the date of the post, though three current employees said the policy began in June.
Four staff researchers, including senior scientist Margaret Mitchell, who was on the research team with Gebru, stated they fear Google is starting to interfere with crucial studies of potential technology harms. “If we are researching the appropriate thing given our expertise, and we are not permitted to publish that on grounds that are not in line with high-quality peer review, then we’re getting into a serious problem of censorship,” stated Mitchell.
Google researchers have published more than 200 papers in the last year about developing AI responsibly, among more than 1,000 projects in total, stated Google Senior Vice President Jeff Dean. Studying Google services for biases is among the “sensitive topics” under the company’s new policy, according to an internal webpage. Among dozens of other “sensitive topics” listed were the oil industry, China, Iran, Israel, COVID-19, home security, insurance, location data, religion, self-driving vehicles, telecoms, and systems that recommend or personalize web content.
Privacy Concerns with Large Language Models as Well
Another issue recently surfaced about large language models is that they run the risk of exposing personal information. Described on Google’s AI blog, the new study was jointly published by Google, Apple, Stanford University, OpenAI, the University of California, Berkeley, and Northeastern University.
Entitled, “Extracting Training Data from Large Language Models,” the new study says the models have the potential to “leak details” from the data on which they are trained. “They can sometimes contain sensitive data, including personally identifiable information (PII) — names, phone numbers, addresses, etc., even if trained on public data,” the study’s authors state.
Calling it a “training data extraction attack,” it has the greatest potential for harm when applied to a model available to the public, but for which the dataset used to train is not. The study authors mounted a proof of concept training data extraction attack on GPT-2, the publicly-available language model developed by OpenAI that was trained using only public data. The results apply to understanding what privacy threats are possible on large language models generally, the authors state.
“The goal of a training data extraction attack is then to sift through the millions of output sequences from the language model and predict which text is memorized,” stated author Nicholas Carlini, Scientist at Google Research. This is a problem because the memorized text may contain someone’s credit card number, for instance.
Results showed that out of 1,800 candidate sequences from the GPT-2 language model, the researchers extracted over 600 that were memorized from the public training data. The memorized examples cover a wide range of content, including news headlines, log messages, JavaScript code, PII, and more.
“While we demonstrate these attacks on GPT-2 specifically, they show potential flaws in all large generative language models,” Carlini stated. “The fact that these attacks are possible has important consequences for the future of machine learning research using these types of models”.
The OpenAI Consortium, whose professed mission is to ensure that AI technology “benefits all of humanity,” released the GPT-2 large language model in February 2019. It was trained on 40Gb of text data and had 1.5 billion parameters.
OpenAI released the GPLT-3 large language model in June 2020. It was trained on 175 billion parameters, 10 times more than the next largest language model, the Turing Natural Language Generation, developed by Microsoft with 17 billion parameters, according to an article explaining the GPT-3 large language model posted on the website of Sigmoid, a company that operates and manages data platforms.
The ability of the GPT-2 model to generate fake news became controversial. “The fake news generated by GPT-3 has been so difficult to distinguish from the real ones, and in one of the experiments, the results show that only 50% of the fake news could actually be detected!” stated Bhaskar Ammu, Senior Data Scientist at Sigmoid who authors the article. He specializes in designing data science solutions for clients, building database architectures and managing projects and teams.
Unlike many language models, GPT-3 does not need Transfer Learning, where the model is fine-tuned on task specific data sets for specific tasks. “The applications of GPT-3 are in-context learning, where a model is fed with a task/prompt/shot or an example, and it responds to it on the basis of the skills and pattern recognition abilities that were learned during the training to adapt the current specific task,” he stated.
“Despite its tremendous usability, the huge model size is the biggest factor hindering the usage for most people, except those with available resources,” Ammu stated. “However, there are discussions in the fraternity that distillation might come to the rescue.”
Read the source articles in VentureBeat, Reuters, on Google’s AI blog, in the paper “Extracting Training Data from Large Language Models, and an article explaining the GPT-3 large language model posted on the website of Sigmoid.