Orchestrating data for machine learning pipelines
Machine learning (ML) workloads require efficient infrastructure to yield rapid results. Model training relies heavily on large data sets. Funneling this data from storage to the training cluster is the first step of any ML workflow, which significantly impacts the efficiency of model training.Data and AI platform engineers have long been concerned with managing data with these questions in mind: Data accessibility: How to make training data accessible when data spans multiple sources and data is stored remotely? Data pipelining: How to manage data as a pipeline that continuously feeds data into the training workflow without waiting? Performance and GPU utilization: How to achieve both low metadata latency and high data throughput to keep the GPUs busy? This article will discuss a new solution to orchestrating data for end-to-end machine learning pipelines that addresses the above questions. I will outline common challenges and pitfalls, followed by proposing a new technique, data orchestration, to optimize the data pipeline for machine learning.To read this article in full, please click here
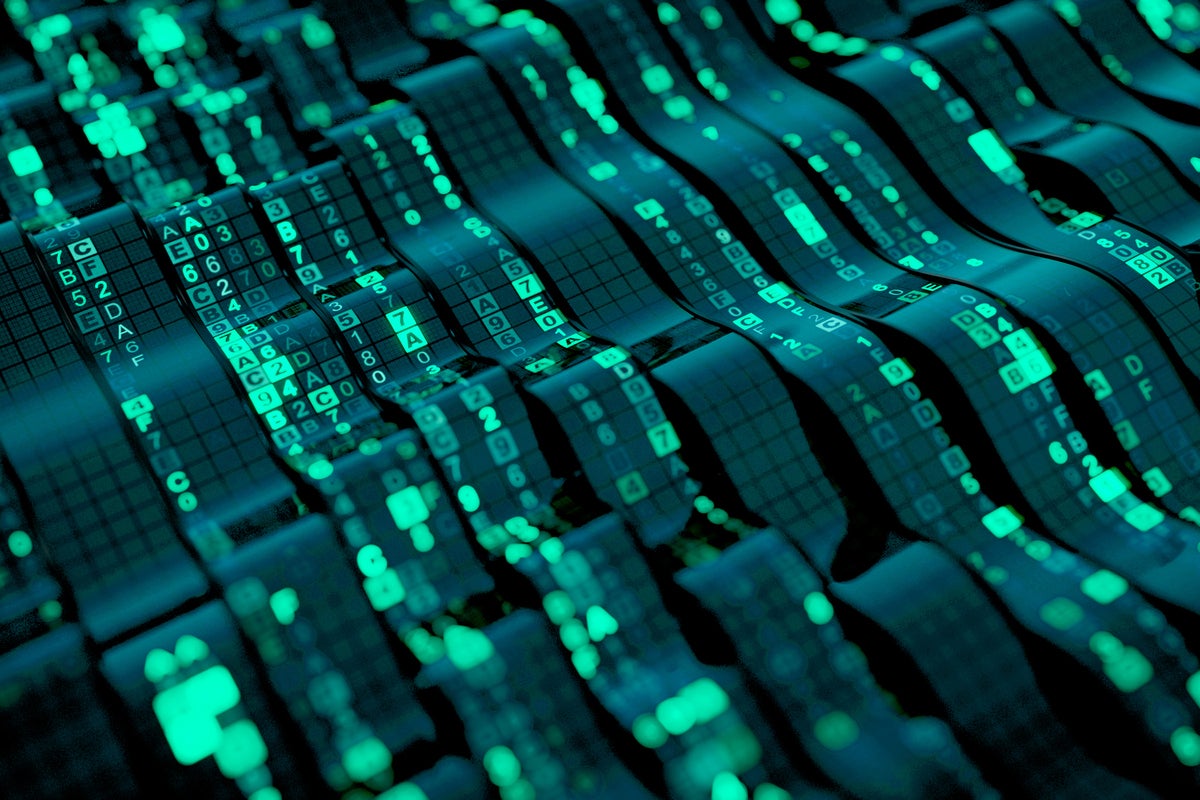
Machine learning (ML) workloads require efficient infrastructure to yield rapid results. Model training relies heavily on large data sets. Funneling this data from storage to the training cluster is the first step of any ML workflow, which significantly impacts the efficiency of model training.
Data and AI platform engineers have long been concerned with managing data with these questions in mind:
- Data accessibility: How to make training data accessible when data spans multiple sources and data is stored remotely?
- Data pipelining: How to manage data as a pipeline that continuously feeds data into the training workflow without waiting?
- Performance and GPU utilization: How to achieve both low metadata latency and high data throughput to keep the GPUs busy?
This article will discuss a new solution to orchestrating data for end-to-end machine learning pipelines that addresses the above questions. I will outline common challenges and pitfalls, followed by proposing a new technique, data orchestration, to optimize the data pipeline for machine learning.