How to Meet the Enterprise-Grade Challenge of Scaling AI
By AI Trends Staff Organizations that have made a commitment to developing AI projects and have experienced some success next face the challenges around successfully scaling the project for the enterprise. To experience all the benefits, the organization needs to align the AI to the business strategy, ensure cross-functional collaboration, invest in the right talent […]
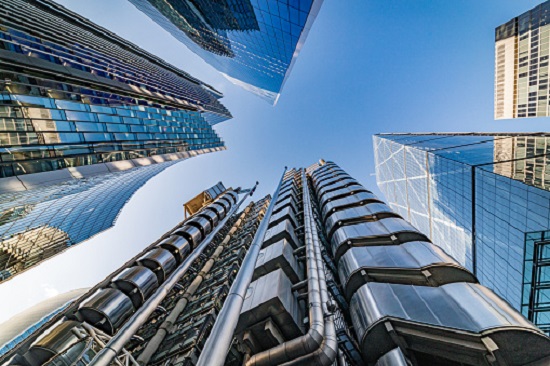
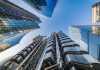
By AI Trends Staff
Organizations that have made a commitment to developing AI projects and have experienced some success next face the challenges around successfully scaling the project for the enterprise.
To experience all the benefits, the organization needs to align the AI to the business strategy, ensure cross-functional collaboration, invest in the right talent and training, and apply strong data practices, suggests a recent account in Tech Wire.
These are no small tasks. A recent global survey on AI conducted by McKinsey found that most respondents who have committed to AI are gaining value, but some are attaining great scale, revenue increases and cost savings than the rest.
A separate survey by Accenture found that companies that strategically scale AI generate five times the return on investment compared to companies that are not able to scale. Some 86% of executives reported that they do not expect to achieve their growth objectives unless they can scale their AI. Furthermore, three-quarters of the C-level executives surveyed believe their companies are likely to go out of business if they fail to aggressively deploy AI throughout their organization.
For some context, McKinsey estimates that AI will add $13 trillion to the global economy in the next decade. The full value of AI can only materialize when firms have offset their upfront costs of development AI, with substantial business gains from its widespread deployment. However, “Most companies are struggling to scale AI,” the account states.
The main reasons that scaling AI is so challenging falls under four themes: customization, data, talent and trust, suggests the writer of a recent account in VentureBeat.
Customization: Most of the models for solving AI problems—ML, deep learning and natural language processing for example—are open sourced, freely available to anyone. Enterprise teams need to customize and train each model to fit the specific problem, data and domain. The model parameters need to be optimized to align to the key performance indicators of the business. To be deployed, the models need to be integrated into the existing IT architecture.
“Building AI systems from scratch for every problem and domain thus requires a ton of customization work,” stated the author, Ganesh Padmanabhan is VP, Global Business Development & Strategic Partnerships at BeyondMinds. Based in Tel Aviv, the company supplies a modular AI engine aimed at solving real-world business problems. “A key part of operationalizing AI is making the customization process as efficient as possible,” he stated.
Data: The effort needed to harness, prepare and access the data to drive AI projects is often underestimated, and is the reason many AI projects fail. In many cases, the organization realizes that they lack standardized data definitions or proper data definitions, and they struggle with distributed data sources. “This kicks off a multi-year transformation journey,” Padmanabhan stated. Advanced machine learning techniques to work with smaller data sets and noisier data in production are needed to get the AI pilot projects to production.
Talent: ML engineers and data scientists who combine statistical (ML) skills, domain expertise and software development experience. “The need to ramp up a team delays your value realization with AI,” he stated, adding, “It takes years for these teams to start producing real results.” Some organizations augment internal AI teams with external partners, for a faster pilot-to-production path, he suggested.
Trust: Given fears AI may make jobs obsolete, AI systems need to be designed with human-machine collaboration at the foundation. “For large-scale adoption of AI across an organization, you need buy-in, support and integration across multiple business processes, IT systems and stakeholder workflows,” Padmanabhan stated.
Maintaining compliance with internal audit and regulatory requirements is a fast-evolving area, also required. Any biased decisions made by black box AI can pose a risk. “This is a critical obstacle that even the most advanced teams will run into when trying to scale AI across their organizations,” he said.
“Siloed Work Culture” Around Data Management Needs to Go
Part of the effort to scale AI in the enterprise may require a transformation of a “siloed work culture,” especially around data management, suggests the founder of a company that helps companies accelerate the adoption of AI.
“Scaling AI in enterprises requires coming together of business, technology and data,” stated Sumanth Vakada, founder and CEO of Qualetics Data Machines, based in Skillman, NJ, in a blog post. “The organizational data needs to be unlocked to ensure its free flow across the organization. This cannot happen in a siloed work culture and organizations must build an interdisciplinary team to drive AI in the organizations,” he suggests.
The effort needs to combine multiple data streams from work teams, applications, clients, products and services. “Each of these areas is capable of generating data that has an impact on other areas laterally,” Vakada stated, adding that the hurdle needs to be overcome to leverage cross-functional data.
If not in place, the organization trying to scale AI needs an “AI Governance Model,” with buy-in from the C-suite, alignment with the business strategy, and structuring of role and responsibilities for execution. One efficient approach is a “hub and spoke: model with the hub taking responsibility for strategy and planning, and small teams in various departments handling execution, he suggests.
“Scaling AI today gives organizations a huge head start not only in picking the low-lying fruits of automation and intelligence, but also in building capacities for the future,” Vakada stated.
Read the source articles and information in Tech Wire, in reports from McKinsey and Accenture, in VentureBeat and in the blog post from Qualetics Data Machines.