How AI Can Live Up to the Hype
Contributed commentary by Roey Mechrez, CTO and Co-founder of BeyondMinds. While we see a lot of hype around AI, the industry is capable of living up to its potential. Here’s the problem: Global spending on AI is widely forecast by IDC analysts to double over the next four years, from $50.1 billion in 2020 to more than $110 billion in 2024. However, […]
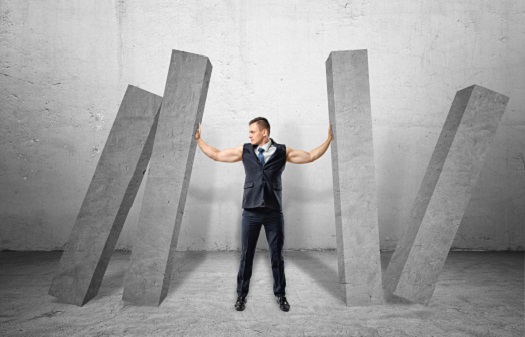
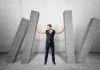
Contributed commentary by Roey Mechrez, CTO and Co-founder of BeyondMinds.
While we see a lot of hype around AI, the industry is capable of living up to its potential.
Here’s the problem: Global spending on AI is widely forecast by IDC analysts to double over the next four years, from $50.1 billion in 2020 to more than $110 billion in 2024. However, much of the money does not yield a return: Some 87% of AI projects fail to even reach production, according to a 2019 account in VentureBeat. That’s a lot of wasted investment.
How do we bridge the gap so that reality meets the high hopes?
As the founder of a company that provides AI solutions, I believe that AI can go beyond “nice to have” solutions and offer genuinely positive disruption and increased profitability to whole industries. But productizing new mission-critical abilities requires significant effort in building AI systems, and a single model cannot deliver on this. The bar for successful delivery and profitability is much higher.
This discussion around the challenges of AI adoption by enterprises tends to focus on strategy-related barriers, such as lack of talent, difficulty detecting the right problems, and access to data. But these are actually being gradually solved in recent years. The next wave of challenges revolves around the effort it takes to graduate from a ‘nice-to-have’ AI solution to a game-changing one.
This feat requires organizational and technological maturity and solving core algorithmics. Overcoming these new challenges requires systematic investment in what I call the Four Pillars of AI Success:
- Moving away from POCs. POCs are a trap. The proof-of-concept (POC) environment is different from the production environment. In the POC environment, the data is static, clean, and unlimited, there is no regulation or compliance and monitoring is irrelevant. In such a lab environment, high accuracy is usually within reach. But that is not the real world. Rather than wasting time and resources, teams should develop the AI in a production environment, processing real data, drilling down on the actual need and adapting to production dynamics. A production-grade mindset can help immensely, even in early development stages.
- Building for stability and robustness. As AI solutions are heavily dependent on data, they tend to become unstable when data shifts or when noise appears. Stability and robustness are among the critical elements of an AI system that differ from lab to production. Achieving and then maintaining stability in production is a complex undertaking, requiring an understanding of the system’s data inputs, controlling the model feed, and detecting anomalies concerning the training data. Focus on these.
- Improving the models over time with a feedback loop and continual learning. Data constantly changes. This impacts the AI models as they intensively use data, mapping input data to predictions, decisions, and actions. When the data changes, so too should the model. This is why maintaining an AI solution is like shooting a moving target, where the target is data. Acquiring new data in production, collecting user feedback, and continually improving the model over time are all vital to keeping the solution “on the rails” and reducing maintenance costs.
- Monitoring as a means of production. Trustful collaboration between humans and machines is key for the profitability and success of AI applications. Human feedback and understanding of what is happening over time with the ‘machine’ is critical to the automation journey. It is crucial to monitor the data flowing into the system as well as the predictions and the system uncertainty over time, and intervene when necessary. The key elements of monitoring include detecting out-of-distribution samples, understanding data drifts, and identifying cases where the data changes in production. These should trigger alerts requesting human intervention and recommending changes and adaptation.
By investing in the fundamentals of AI systems and deploying these four pillars, a company can plan for successful AI adoption across the organization. Put another way: the industry should move from AI models developed by a researcher to an AI system developed by a diverse team, including many AI components that together assemble a robust system.
The potential of AI is immense and undeniable, which is why we have so much hype around it. Now it’s time to do the hard work needed in order to fulfill the potential.
Roey Mechrez, PhD is CTO and Co-founder of BeyondMinds.