Deep Learning Models Have Advantages for Neuroscience, Researchers Find
By John P. Desmond, AI Trends Editor Neuroscientists using AI models to simulate the brain are learning more about how the brain works and improving AI models. Deep learning (DL) models have advantages over standard machine learning in brain research, according to findings of researchers at Georgia State University recently published in Nature Communications. “Our findings highlight the presence […]
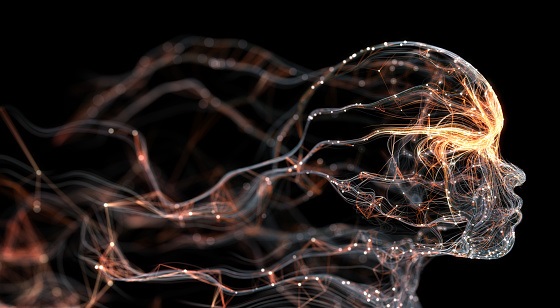
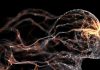
By John P. Desmond, AI Trends Editor
Neuroscientists using AI models to simulate the brain are learning more about how the brain works and improving AI models. Deep learning (DL) models have advantages over standard machine learning in brain research, according to findings of researchers at Georgia State University recently published in Nature Communications.
“Our findings highlight the presence of nonlinearities in neuroimaging data that DL can exploit to generate superior task-discriminative representations for characterizing the human brain,” stated the paper’s lead author Anees Abrol along with Sergey Plis, Vince Calhoun, Yuhui Du, Rogers Silva, Mustafa Salman, and Zening Fu.
In standard machine learning, predictions are a result of processing functions via inference rules. The decision boundaries are determined in the input spaces, which according to the research, is a limiting factor in projects requiring the modeling of complex brain data, according to an account in Psychology Today.
Deep learning has the capability of learning representations, and can learn from the data with minimal or no preliminary preprocessing step. Deep learning has a design that is somewhat inspired by the human brain. The depth in deep learning refers to the many hidden layers of algorithms in between the input and output layer in its artificial neural network. The neural network layers contain computational nodes that are analogous to biological neurons.
The researchers compared the performance of multiple classification and regression tasks between standard machine learning and deep learning approaches. They used MRI data from over 12,000 subjects from the UK Biobank and over 800 subjects from the Alzheimer’s Disease Neuroimaging Initiative (ADNI) repository.
“Results show that if trained following prevalent DL practices, DL methods have the potential to scale particularly well and substantially improve compared to SML methods, while also presenting a lower asymptotic complexity in relative computational time, despite being more complex,” the researchers stated.
Brain Activity in Mice Shows Similarities to Reinforcement Learning Models
Elsewhere, recent research from a joint effort of Harvard University and DeepMind has found properties in the brains of mice that are very similar to those of reinforcement learning models, according to an account in TechTalks. The researchers measured the firing rates of dopamine, a neurotransmitter in the brain that plays a role in how we feel pleasure, to examine the variance in reward prediction rates of biological neurons.
The researchers found similarities between the reinforcement learning models they had programmed to the nervous systems of mice. “We found that dopamine neurons in the brain were each tuned to different levels of pessimism or optimism,” DeepMind’s researchers wrote in a blog post published on the lab’s website. “In artificial reinforcement learning systems, this diverse tuning creates a richer training signal that greatly speeds learning in neural networks, and we speculate that the brain might use it for the same reason.”
They added, “It gives us increased confidence that AI research is on the right track, since this algorithm is already being used in the most intelligent entity we’re aware of: the brain.”
German Researchers Find Single Neurons Can Perform XOR Functions
Researchers in Berlin, in a study published in Science in January, found that some fundamental assumptions made about the brain are wrong. The German researchers found that single neurons can perform XOR functions, which compare two input bits and generate one output bit. This premise was rejected by AI pioneers including Marvin Minsky and Seymour Papert, authors of the book Perceptrons, published in 1969, which argued that a single neuron could not perform an XOR function. The effect was to put a damper on the study of neural networks for many years.
Konrad Kording, a computational neuroscientist at the University of Pennsylvania who was not involved in the research, told Quanta Magazine that the finding could mean “a single neuron may be able to compute truly complex functions. For example, it might, by itself, be able to recognize an object.”
The suggestion is that scientists may need to rethink the modeling of neurons. It could spur research in new artificial neuron structures and networks with different types of neurons.
Salk Institute Researchers Study Technique for AI to Learn Faster
Research at the Salk Institute is providing insight into how to get computers to think more like humans. The researchers used a computational model of brain activity to mimic how the brain’s prefrontal cortex uses a phenomenon known as “gating” to control the flow of information between different areas of neurons, according to an account in Neuroscience News.
The finding could inform the design of new AI programs. “If we can scale this model up to be used in more complex artificial intelligence systems, it might allow these systems to learn things faster or find new solutions to problems,” stated Terrence Sejnowski, head of Salk’s Computational Neurobiology Laboratory and senior author of the new work, published on November 24, 2020, in Proceedings of the National Academy of Sciences.
The new network performed as reliably as humans on the Wisconsin Card Sorting Task, a cognitive test. It also mimicked the mistakes seen in some patients. When sections of the model were removed, the system showed the same errors seen in patients with prefrontal cortex damage, such as that caused by trauma or dementia.
“One of the most exciting parts of this is that, using this sort of modeling framework, we’re getting a better idea of how the brain is organized,” stated Ben Tsuda, a Salk graduate student and first author of the new paper. “That has implications for both machine learning and gaining a better understanding of some of these diseases that affect the prefrontal cortex.”
The team next wants to scale up the network to perform more complex tasks than the card-sorting test and determine whether the network-wide gating gives the artificial prefrontal cortex a better working memory in all situations.
Read the source articles in Nature Communications, Psychology Today, TechTalks and Neuroscience News.