Best practices for developing governable AI
Building and deploying strong, robust artificial intelligence (AI) and machine learning (ML) models is complex and challenging work. If you are like many data science and machine learning leaders that I have spoken to lately, you are having conversations with other teams about the governance of your systems.It’s hard to do that and do your job of getting models into production. So let’s talk about what you can do as a technical organization to make AI governance easier both for your team and your business partners, who are key stakeholders in the governance process. [ Also on InfoWorld: How to choose a cloud machine learning platform ] Key design principles At a high level, to ensure that we have models that are governable and can be assured, we want to make sure model artifacts exhibit the following three principles:To read this article in full, please click here
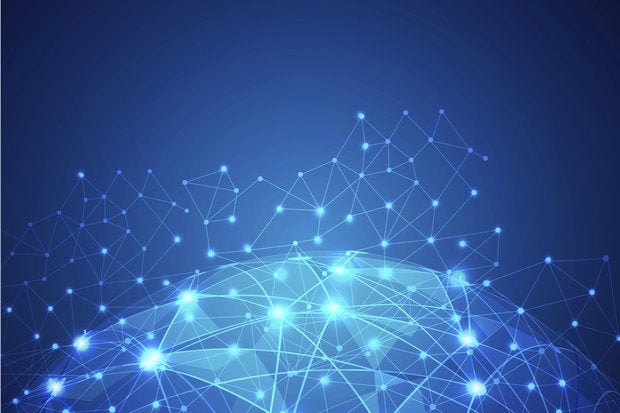
Building and deploying strong, robust artificial intelligence (AI) and machine learning (ML) models is complex and challenging work. If you are like many data science and machine learning leaders that I have spoken to lately, you are having conversations with other teams about the governance of your systems.
It’s hard to do that and do your job of getting models into production. So let’s talk about what you can do as a technical organization to make AI governance easier both for your team and your business partners, who are key stakeholders in the governance process.
Key design principles
At a high level, to ensure that we have models that are governable and can be assured, we want to make sure model artifacts exhibit the following three principles: